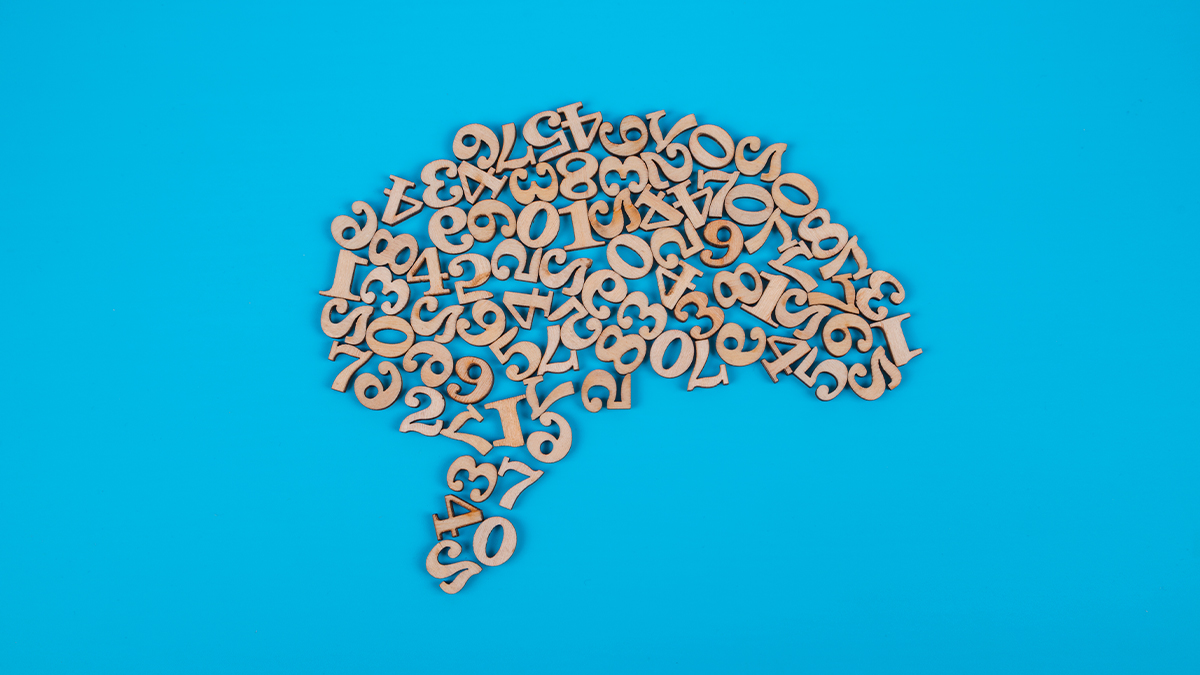
Big data projects that revolve around exploiting data for business optimization and business development are top of mind for most executives. However, up to 85% of big data projects fail, often because executives cannot accurately assess project risks at the outset. We argue that the success of data projects is largely determined by four important components — data, autonomy, technology, and accountability — or, simply put, by the four D.A.T.A. questions. These questions originate from our four-year research project on big data commercialization.
The components needed for success with big data can be positioned along two dimensions: (1) the focus of the activities (the project’s ideation or implementation — such as coming up with an idea for a big data project versus actually implementing the project) and (2) the focus of the transformation (digital backbone or getting people’s support — such as building the IT-architecture needed to create a sufficient digital backbone or making sure that employees can and will apply data and that this application is in line with societal opinions on what should and should not be done with data). These two dimensions create a matrix of D.A.T.A. components and the key questions executives need to ask when contemplating new big data projects, as seen below.
Table of Contents
The D.A.T.A. Questions You Need to Ask
The figures below provide an overview of the D.A.T.A. components, the related questions, and the rationale behind them as well as examples. Moreover, the table demonstrates the sequence in which the different aspects should be considered in data projects. In the following, we provide an in-depth outline of each component.
Data: Access to data is obviously a precondition for any initiative focused on data-driven growth. However, not all available data is useful, nor is it unique and exclusive. Moreover, not all data is available. The question executives need to ask is: “Can we access data that is valuable and rare?” Only when these criteria are fulfilled can executives hope to gain a temporary competitive advantage based on data.
Consider the Danish social fitness-tracking app Endomondo. In 2015, the American athletic apparel company Under Armor bought Endomondo for $85 million in an attempt to build “the world’s largest digital health and fitness community.” Endomondo had more than 20 million users and over 80% of them were located outside the United States. Hence, Under Armour expected the acquisition to not only provide access to data that it considered valuable and rare, but also to give the company “immediate scale and increased international presence,” as stated in the press release. The very price that Under Armor was willing to pay illustrates the value that it assigned to Endomondo’s data. Consequently, data is becoming a resource that increases in commercial potential with its user value and its uniqueness in the marketplace.
Autonomy: Autonomy, or decentralized decision-making, gives employees a mandate to come up with ideas for data-enabled solutions that they can initiate on their own. It is an essential step in the ideation phase, and it similarly deals with the human component of digital transformations. In this regard, the question executives need to ask is: “Can employees use data to create solutions on their own?” Unlocking the commercial potential of data requires the engagement of many people from multiple levels and departments.
Google serves as an example of this key component. Google has long been famous for allowing decentralized decision-making and for making dedicated resources available for initiatives across the organization. Google has “20% time,” where it allows engineers to work on projects of their own choosing 20% of the time. Moreover, Google makes its computing resources and data available to its engineers’ 20% projects. One project that originated as a result of the 20% time policy and that made extensive use of a variety of data was “Google Now” (a mobile assistant that proactively delivered information to users based on search habits). Although Google Now no longer exists, the underlying functionality is still used. Autonomy is not only important for creating and launching new initiatives, but also for learning and for adapting existing processes. Consequently, employees need to be able to use data to initiate, create, and adapt their own solutions.
Technology: Technology is similarly essential for success with data. It comprises an important first step in the implementation phase as well as an essential component for the digital backbone. Here, the question executives need to ask is: “Can our technology deliver the solution?” You can have all the data and ideas in the world, but if your technology can only deliver a prototype, a beta version or non-scalable volume, no real value will ultimately be created for your firm.
An example of the importance of technology is illustrated in the blockchain collaboration between Maersk and IBM. Maersk had long wanted to streamline and simplify the administrative hurdles of global trade. However, it was unable to do so before the emergence of blockchain, which provided the technical backbone to create a solution to the problem. As Maersk arguably did not have the needed blockchain capabilities in-house, it partnered with IBM to create Tradelens, a global trade platform designed to minimize costs and increase the transparency of global shipping. In other words, enabled by blockchain technology, Tradelens digitizes the global supply chain and provides information to all actors. Consequently, it is necessary for any data-enabled solution to have sufficient technology to deliver it.
Accountability: Accountability refers to obtaining both regulatory and societal permission for your data solution as well as being able to demonstrate this compliance. It comprises an important step in the implementation phase and is an essential part of the human component of digital transformations. The question executives need to ask in this regard is the following: “Is our solution compliant with laws and ethics?” Little value can be created if your solution breaks the law. Moreover, if users think of the solution as “creepy,” you might face a media backlash.
The impacts of neglecting accountability can be seen in the Facebook/Cambridge Analytica scandal. As shown in the Netflix documentary “The Great Hack,” Cambridge Analytica harvested personal Facebook data to identify and target “persuadables” (i.e., people likely to be persuaded by certain messages) with the aim of changing their minds in the 2016 U.S. presidential election. The argued misuse of personal data raised questions from both legal and ethical points of view. The case has had severe implications for the parties involved, and it has made both users and lawmakers more aware of the legal and ethical challenges of data collection and usage. Consequently, no solution should be expected to become a long-term success if it is not compliant with the law, societal ethics, and norms.
Scoring your next big data project
As you have probably noticed, these D.A.T.A. questions ask for simple yes/no answers. This means that you can determine the likelihood that your next big data project will be a success by tallying your answers to the questions. In other words, if you answer “yes” to three questions and “no” to one, your project has a score of 3.
Use the table below to score your next project. As is evident from the table, you will need a score of 4 to be confident of success with your data project. A score of 3 means that the project still requires substantial work in order to succeed, as all four components are essential for big data projects. Consequently, you can think of the components as need to have rather than nice to have.
Scoring Your Big Data Project
You can determine the likelihood that your next big data project will be a success by tallying your answers to the D.A.T.A. key questions. Each “yes” answer scores one point.
Score | Meaning |
4 | Your data project has a high likelihood of success. |
3 | Your data project needs critical work to achieve success. |
2 | Your data project is unlikely to achieve success. |
1 | Your data project is very unlikely to achieve success. |
0 | Your data project should be terminated immediately. |
Source: Carsten Lund Pedersen and Thomas Ritter | © HBR.org |
An example illustrates the necessity of all four components. Facebook has unique and valuable personal data, such as data on users’ relationship status. In fact, Facebook is able to accurately predict whether a given relationship will last. While this may comprise unique and valuable data (“yes” to question 1) that Facebook employees are free to build solutions around (“yes” to question 2), and even though Facebook has the technology to deliver such a solution (“yes” to question 3), such a solution would seem slightly unethical to most people (“no” to question 4). Therefore, such a project would be assigned a score of 3, as critical work on accountability is required for it to be successful.
Big data projects are arguably in need of a more data-driven approach to predicting their success. While expectations regarding the business potential of big data are astronomical, returns have thus far been weak. Part of the problem is arguably that executives do not know how to predict the success of data projects, as few structured approaches to doing so exist. With our D.A.T.A. framework, executives can finally start to make data-informed decisions on big data projects.