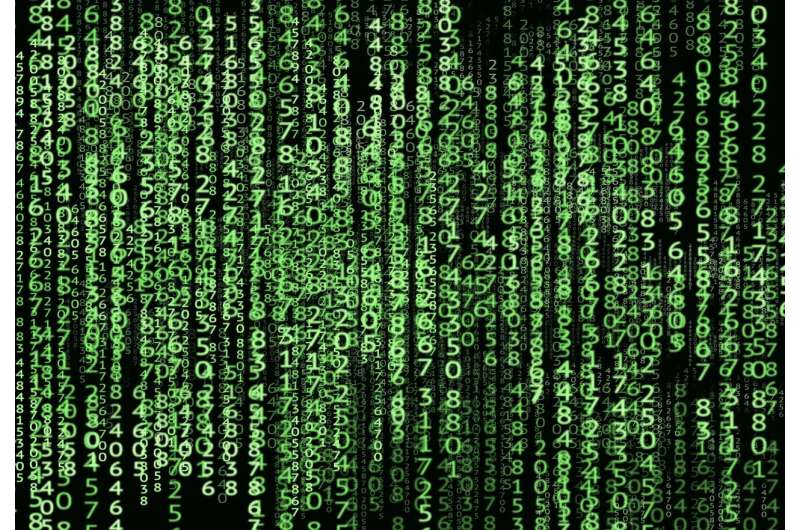
A huge volume of digital data has been harvested, stored and shared in the last few years from sources such as social media, geolocation systems and aerial images from drones and satellites, giving researchers many new ways to study information and decrypt our world. In Switzerland, the Federal Statistical Office (FSO) has taken an interest in the big data revolution and the possibilities it offers to generate predictive statistics for the benefit of society.
Conventional methods such as censuses and surveys remain the benchmark for generating socio-economic indicators at the municipal, cantonal and national levels. But these methods can now be supplemented with secondary, mostly pre-existing data, from sources such as cell-phone subscriptions and credit cards. According to the FSO’s 2017 Data Innovation Strategy, “The goal of data innovation is to enhance the quality, scope and cost-efficiency of statistical products and to reduce the response burden on households and businesses.”
Anonymized data
Against this backdrop, a team of scientists at EPFL’s Laboratory on Human-Environment Relations in Urban Systems (HERUS) conducted a ground-breaking study on novel uses for the data held by insurance companies. The lab’s leading partner company, La Mobilière, provided anonymized data from hundreds of thousands of policyholders. These data included factors such as age, residential postal code, car- and homeownership, and employment status.
“We wanted to see if we could use these data to predict specific socio-economic indicators—ones that could give us a better picture of the quality of Switzerland’s urban areas. One big advantage of the data held by insurers—provided they’re willing to share it—is that they are cheap to use, since they already exist, and annual surveys can be carried out at no extra cost,” says Emanuele Massaro, a lead author of the study, which was published in PLOS ONE on 3 March.
Using data-mining techniques, the research team extracted the relevant information and aggregated it to cover the 170 most populated Swiss towns. In all, they obtained nearly 600,000 profiles, each identified by a unique code. “La Mobilière’s dataset is very complete; it contains a wide range of information that enabled us to factor in over 30 variables, which we used mainly to select those variables that best match each socio-economic indicator,” says Lorenzo Donadio, a Master’s student in environmental science and engineering at EPFL and the study’s first author.
A spatial regression model
The scientists developed a spatial regression model to accurately predict twelve variables in six categories: population, transport, work, space and region, housing, and the economy. “Of course, our predictions can’t replace official censuses, but they can serve as yearly signposts. We also wanted to show that insurers’ datasets contain a great deal of socially relevant information—beyond what they use for marketing and market research—and that insurers should consider working more closely with researchers,” says Massaro.
The team’s statistical model was developed solely for research purposes and has no practical application as such. It could be used to help guide policymakers, but regular census data are still needed. La Mobilière’s data are missing certain information, such as for young people under the age of 18, but are nevertheless representative of a large portion of the population. “Our model could be used by city policymakers and government statistical offices, which could incorporate this type of information in their modernization efforts. Insurers’ datasets are highly granular because they contain very specific information about their customers,” says Massaro.
Swiss statistical systems enhanced by big data (2021, March 3)
retrieved 3 March 2021
from https://phys.org/news/2021-03-swiss-statistical-big.html
part may be reproduced without the written permission. The content is provided for information purposes only.
This content was originally published here.