Machines not only understand but also create art, music and literature. This is the world we are entering, where AI reigns supreme. It’s like AI Picasso, Mozart and Shakespeare rolled into one.
So, what’s the buzz about generative AI (generative artificial intelligence)? Well, it’s not just another fancy term in the AI universe. It’s a game-changer, a disruptor, and a muse for the digital age. In this article, we’re going to unravel the mysteries behind generative AI — what it is, how it works, and why it’s causing such a stir in the tech world and beyond.
Table of Contents
What Is Generative AI’s Meaning?
Generative AI is actually like having a virtual artist, composer, and storyteller all rolled into one. It’s the subset of artificial intelligence focused on autonomously creating new content – images, text, music, and even entire scenarios. Unlike traditional AI, which follows predefined rules, generative AI relies on advanced algorithms and deep learning techniques to mimic and often surpass human-like creativity.
How Does Generative AI Work?
- Intricate Neural Networks:
Generative AI operates through complex neural networks, inspired by the structure of the human brain.
- Training on Vast Datasets:
These networks are trained on vast datasets, learning to recognize patterns and relationships within the data.
- Generative Modeling:
Through a process called generative modeling, they extrapolate from these patterns to create new content autonomously.
Generative Adversarial Networks (GANs)
- Two Neural Networks:
GANs consist of two neural networks—the generator and the discriminator—that work in tandem.
- Generating Content:
The generator creates new content, such as images or text, while the discriminator evaluates the authenticity of the generated content.
- Iterative Learning:
Through repeated iterations, the generator learns to produce increasingly realistic output, while the discriminator becomes more adept at distinguishing between real and fake data.
Impact of Generative AI
- Generative AI, particularly through techniques like GANs, is revolutionizing the way we create and interact with digital content.
- As these technologies continue to evolve and improve, the possibilities for innovation and creativity are truly limitless.
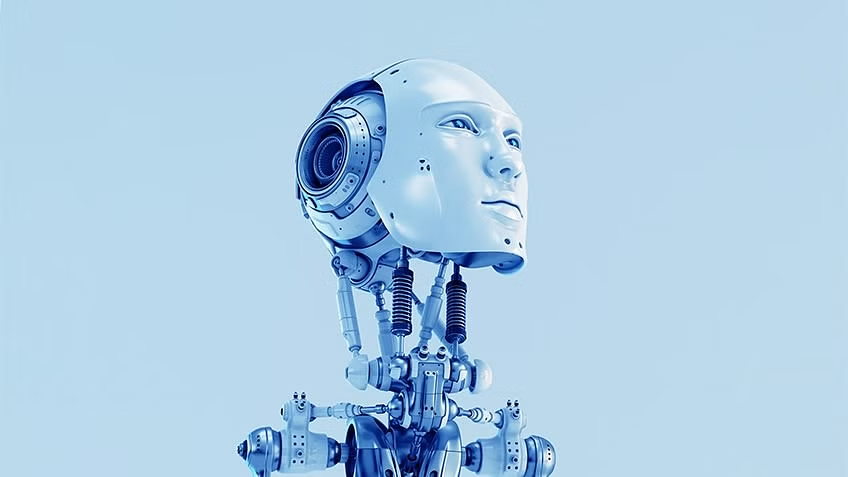
What are the Benefits of Applying Generative AI?
Generative AI stands at the forefront of technological innovation, promising a multitude of benefits across diverse industries. These benefits are:
- Enhanced Creativity:
Generative AI unlocks new levels of creativity by autonomously generating content, ranging from images and text to music and beyond. This not only streamlines the creative process but also inspires innovation and experimentation.
- Efficiency in Design:
For artists and designers, generative AI tools serve as invaluable aids, offering endless inspiration and new ideas, streamlining the creative process. By automating repetitive tasks such as generating design variations or exploring different aesthetic possibilities, generative AI allows creators to focus their time and energy on refining and implementing their vision. This leads to more efficient workflows and faster iteration cycles, ultimately resulting in higher-quality designs.
- Innovative Solutions:
In healthcare, generative AI accelerates drug discovery and development, leading to more effective treatments by simulating molecular structures and interactions. By leveraging generative AI algorithms, researchers can explore vast chemical spaces and identify potential drug candidates more efficiently than traditional methods. This not only speeds up the drug discovery process but also reduces costs and minimizes the risk of failure, ultimately improving patient outcomes and saving lives.
According to a report by McKinsey & Company, the use of generative AI in drug discovery has led to a 30% increase in the number of successful drug trials.
- Personalized Content:
In marketing and advertising, generative AI personalizes content and tailors marketing campaigns to individual preferences, increasing engagement and conversion rates. By analyzing customer data and behavior, generative AI can dynamically generate personalized advertisements, product recommendations, and marketing messages tailored to each customer’s interests and preferences. This not only enhances the customer experience but also maximizes the effectiveness of marketing efforts, driving higher ROI for businesses.
Research conducted by Epsilon found that personalized marketing campaigns have an average ROI that is three times higher than generic campaigns.
- Realistic Simulations:
Generative AI creates realistic simulations for training purposes, from flight simulators to medical training scenarios, enhancing learning outcomes and proficiency. By generating highly realistic virtual environments and scenarios, generative AI enables trainees to practice and refine their skills in a safe and controlled setting, without the need for expensive or dangerous real-world experiments. This not only accelerates learning but also improves retention and proficiency, ultimately leading to better-trained professionals across various industries.
- Automation of Repetitive Tasks:
By automating repetitive tasks, generative AI frees up human resources to focus on more complex and strategic initiatives, increasing productivity and efficiency. Whether it’s generating design variations, processing large datasets, or performing routine administrative tasks, generative AI can handle repetitive tasks with speed and accuracy, allowing human workers to focus on higher-value activities that require creativity, critical thinking, and problem-solving skills. This not only improves overall productivity but also enhances job satisfaction and morale among employees.
- Diverse Applications:
Generative AI finds applications in various industries, from architecture and entertainment to fashion and gaming, driving innovation and pushing the boundaries of creativity. Whether it’s generating architectural designs, creating lifelike characters and environments for video games, or designing custom clothing and accessories, generative AI is revolutionizing the way we create and interact with digital content. With its ability to generate novel and imaginative solutions to complex problems, generative AI is poised to play a central role in shaping the future of design, innovation, and creativity across a wide range of industries.
Some Risks Associated with Generative AI
While the potential of generative AI is vast and promising, it also presents certain risks and challenges that need to be carefully considered. Here are some of the key risks associated with generative AI:
- Bias and Fairness:
Generative AI models are trained on large datasets, which may contain inherent biases present in the data. These biases can be perpetuated and amplified by the AI system, leading to biased outcomes and unfair treatment of certain groups. For example, if a generative AI model is trained on data that disproportionately represents one demographic group over others, it may produce biased content that reflects or reinforces these disparities.
According to a study by MIT, biases in generative AI systems can result in up to 25% more bias than in the training data.
- Misuse and Malicious Use:
Generative AI technologies can be used to create convincing fake content, such as Deepfake videos or counterfeit images, with potentially harmful consequences. For example, malicious actors could use generative AI to create fake news articles, impersonate individuals, or spread disinformation campaigns. This poses significant risks to individuals, organizations, and society at large, undermining trust and causing social unrest.
According to a report by the RAND Corporation, the proliferation of Deepfake technology poses a significant threat to national security and democratic institutions.
- Privacy Concerns:
Generative AI models trained on sensitive or personal data may inadvertently disclose confidential information or compromise individuals’ privacy. For example, if a generative AI model is trained on healthcare data to generate synthetic patient records, there is a risk that the generated data could be re-identified or used to infer sensitive information about individuals. This raises concerns about data privacy and security, as well as compliance with regulations such as GDPR and HIPAA.
According to a survey by PwC, 85% of consumers are concerned about the privacy implications of AI technologies. This raises concerns about data privacy and security, as well as compliance with regulations such as GDPR and HIPAA.
- Intellectual Property Issues:
Generative AI models can generate content that may infringe upon existing intellectual property rights, such as copyrights, trademarks, or patents. For example, if a generative AI model is trained on copyrighted texts or images, it may produce derivative works that violate the original creators’ rights. This raises legal and ethical questions about ownership, attribution, and compensation for generated content.
According to a study by Stanford University, the use of generative AI for content creation raises complex legal and ethical questions regarding intellectual property ownership and attribution.
- Ethical Dilemmas:
Generative AI raises complex ethical dilemmas regarding its use and impact on society. For example, should generative AI be used to create lifelike avatars of deceased individuals, or to generate realistic simulations of violent or explicit content? How should generative AI be regulated to ensure its responsible and ethical use? These questions require careful consideration and deliberation by policymakers, researchers, and industry stakeholders.
According to a survey by the Pew Research Center, 63% of Americans believe that the use of AI raises ethical concerns. These questions require careful consideration and deliberation by policymakers, researchers, and industry stakeholders.
Addressing these risks and challenges requires a multi-faceted approach, involving collaboration between policymakers, industry leaders, researchers, and ethicists. Developing robust governance frameworks, ethical guidelines, and technical safeguards is essential to mitigate the risks associated with AI consulting services and ensure its responsible and beneficial use for society.
Overview of Generative AI Models
Generative AI encompasses a variety of models and techniques, each with its own strengths and applications. Here are some key types of generative AI models:
- Generative Adversarial Networks (GANs):
GANs consist of two neural networks—the generator and the discriminator—that work in tandem. The generator creates new content, such as images or text, while the discriminator evaluates the authenticity of the generated content. Through iterative training, GANs learn to produce increasingly realistic output, often indistinguishable from human-generated material.
- Variational Autoencoders (VAEs):
VAEs are probabilistic generative models that learn to encode and decode high-dimensional data, such as images or text. Unlike GANs, which generate content through adversarial training, VAEs generate content by learning a latent representation of the data’s underlying distribution. This allows VAEs to generate diverse and realistic samples, albeit with less sharpness and detail compared to GANs.
- Autoregressive Models:
Autoregressive models generate content one element at a time, conditioned on previous elements. Examples include autoregressive language models like GPT (Generative Pre-trained Transformer) and PixelRNN for image generation. These models are highly flexible and can generate coherent and contextually relevant sequences, making them well-suited for tasks such as text generation and image synthesis.
- Flow-Based Models:
Flow-based models learn a bijective mapping between data samples and a latent space, enabling efficient generation and density estimation. Unlike autoregressive models, which generate samples sequentially, flow-based models can generate samples in parallel, making them computationally efficient for high-dimensional data like images.
- Attention-Based Models:
Attention mechanisms allow models to focus on different parts of the input data, enabling more flexible and context-aware generation. Models like the Transformer architecture, used in GPT and BERT (Bidirectional Encoder Representations from Transformers), leverage attention mechanisms to generate coherent and contextually relevant sequences of text.
Each of these generative AI models has its own strengths and weaknesses, and the choice of model depends on the specific task and requirements. By leveraging the capabilities of these models, researchers and practitioners can unlock new possibilities in creative content generation, simulation, and problem-solving.
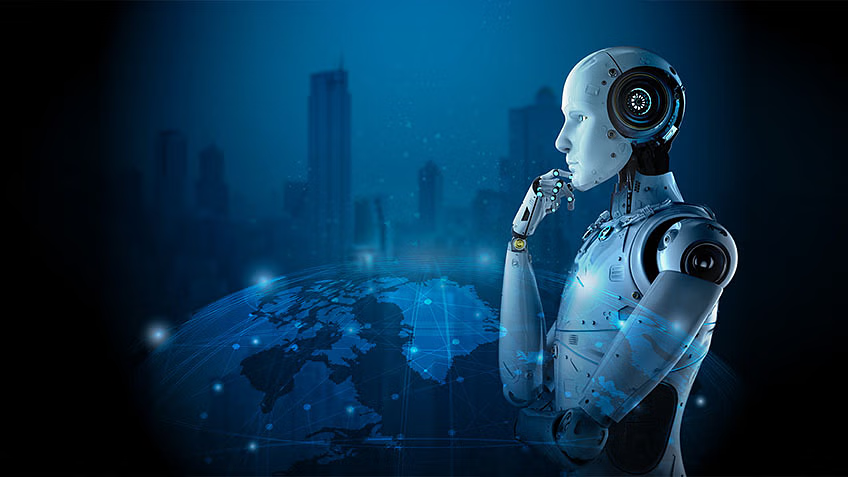
Practical Applications of Generative AI Today
Generative AI finds diverse practical applications across industries, transforming digital content creation, creative design, healthcare, gaming, training, and artificial creativity. Here are some prominent examples:
- Content Creation:
Widely used in generating images, videos, and text for entertainment, advertising, and marketing.
- Creative Design:
Revolutionizing architecture, fashion, and graphic design by generating innovative concepts and designs.
- Healthcare and Medicine:
Enhancing drug discovery, medical imaging, and personalized medicine through simulations and analysis.
- Gaming and Virtual Reality:
Powering lifelike characters, procedurally generated worlds, and immersive experiences in gaming and VR.
- Simulation and Training:
Used in aviation, defense, and education for realistic simulations, training scenarios, and interactive learning.
- Artificial Creativity:
Expanding the boundaries of AI-generated artworks, music composition, and literary creations.
Generative AI’s impact continues to grow, offering new avenues for innovation and creativity while reshaping industries and human-machine interactions.
Frequently Asked Questions
What is Generative AI?
Generative AI refers to the subset of artificial intelligence focused on producing new content autonomously, often surpassing human-like creativity.
What are Some Examples of Generative AI?
Examples of generative AI include image generation, text generation, music composition, and even the creation of entire scenarios or environments.
What Can AI Generate?
AI can generate a wide range of content, including images, text, music, videos, and even entire virtual environments.
In conclusion, generative AI holds incredible promise for the future, offering unprecedented opportunities for creativity, innovation, and advancement across a multitude of fields. However, it is essential to proceed with caution, mindful of the risks and ethical considerations associated with this powerful technology. As we continue to push the boundaries of what is possible with generative AI, it is crucial to ensure that its benefits are harnessed responsibly and ethically, for the betterment of society as a whole.