Artificial Intelligence (AI) has revolutionized many fields, and anomaly detection is no exception. AI anomaly detection has become a critical component in various industries, enabling organizations to identify and address unusual patterns or behaviors that deviate from the norm. In this comprehensive article, we will explore the concept of anomaly detection in AI, its use cases, benefits, common methods, and why Vinova’s AI development services are the best choice for your anomaly detection needs.
Table of Contents
What is Anomaly Detection in AI?
Anomaly detection refers to the identification of items, events, or observations that do not conform to an expected pattern in a dataset. These anomalies can signify critical incidents such as fraud, network intrusions, equipment failures, or other significant issues that require immediate attention.
In the realm of AI, anomaly detection leverages machine learning algorithms and deep learning techniques to automatically discover these irregularities. This process involves training AI models on historical data so they can learn the normal behavior of the system and subsequently detect deviations that could indicate anomalies.
Use Cases of AI in Anomaly Detection
AI anomaly detection has diverse applications across various industries. Below are some notable use cases:
Cybersecurity
In cybersecurity, AI anomaly detection is employed to identify unusual patterns that may indicate a cyberattack or data breach. By continuously monitoring network traffic and user behavior, AI models can detect and respond to threats in real-time, significantly reducing the potential damage caused by malicious activities.
Finance
In the financial sector, AI anomaly detection algorithms are used to detect fraudulent transactions and irregularities in trading patterns. This technology helps banks and financial institutions protect their assets and maintain the trust of their customers by quickly identifying and addressing suspicious activities.
Manufacturing
Manufacturing industries use AI anomaly detectors to monitor equipment and production processes. By identifying anomalies in machine performance or product quality, manufacturers can prevent costly downtime and ensure the consistent production of high-quality goods.
Telecommunications
In telecommunications, time series anomaly detection is crucial for maintaining network reliability and performance. AI algorithms analyze vast amounts of network data to detect and rectify issues such as service outages, network congestion, and other operational anomalies.
Healthcare
Healthcare providers use AI for anomaly detection to monitor patient health data and detect early signs of medical conditions. For example, AI can analyze vital signs and medical records to identify anomalies that may indicate a patient’s deteriorating health, allowing for timely intervention.
Retail
Retail businesses employ anomaly detection machine learning to monitor sales data, inventory levels, and customer behavior. This helps retailers optimize their operations, prevent stockouts or overstock situations, and enhance customer satisfaction by predicting and addressing potential issues.
Benefits of AI in Anomaly Detection
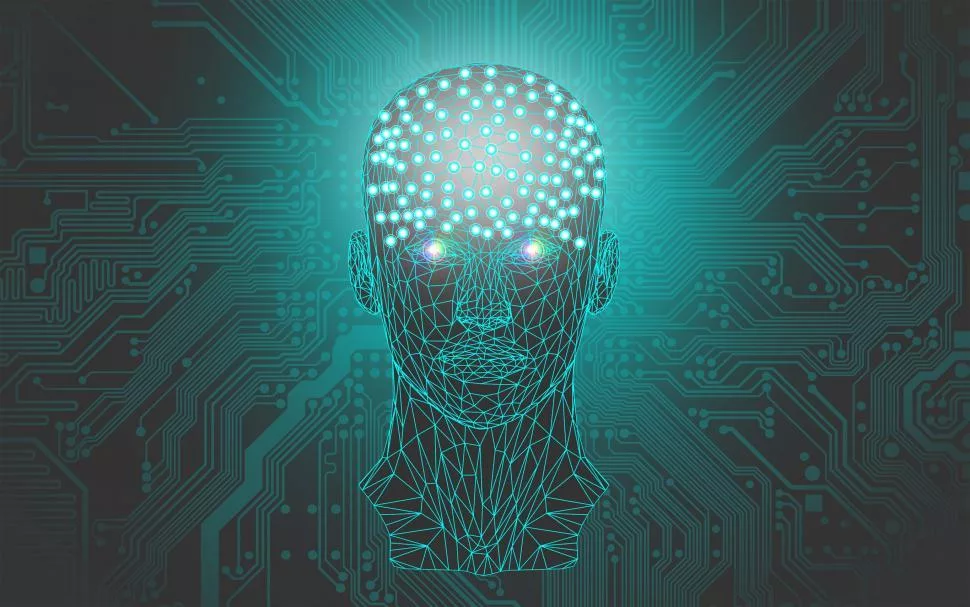
The integration of AI in anomaly detection offers numerous advantages that significantly enhance the ability of organizations to identify and respond to irregularities. Here are some of the key benefits:
Accuracy and Efficiency
AI models are capable of processing and analyzing large volumes of data with high precision. This capability ensures that anomalies are detected accurately and efficiently, often surpassing the performance of traditional methods. The key aspects of this benefit include:
- High Precision: AI algorithms can discern subtle patterns and correlations in data that may not be apparent to human analysts or conventional detection methods. According to a study by Deloitte, AI-powered anomaly detection systems can achieve precision rates of up to 95%, surpassing traditional methods.
- Error Reduction: The accuracy of AI models minimizes false positives (incorrectly identifying normal behavior as anomalous) and false negatives (failing to identify actual anomalies), leading to more reliable detection. Research published in the International Journal of Advanced Computer Science and Applications indicates that AI-driven anomaly detection reduces false positives by 30% and false negatives by 25% compared to rule-based methods.
- Speed: AI systems can analyze data in real-time or near-real-time, enabling the swift identification of anomalies and facilitating rapid response actions.
Real-time Monitoring
AI-powered anomaly detection systems are designed for continuous monitoring, which is crucial for detecting and addressing anomalies as soon as they occur. This real-time capability provides several benefits:
- Instant Alerts: AI systems can generate immediate alerts when an anomaly is detected, ensuring that potential issues are promptly brought to the attention of relevant personnel.
- Proactive Response: Continuous monitoring allows organizations to take proactive measures to mitigate the impact of anomalies before they escalate into more significant problems. A case study by IBM highlighted that organizations using AI for real-time anomaly detection saw a 40% decrease in incident response time, leading to better operational continuity.
- Operational Continuity: Real-time detection helps maintain smooth and uninterrupted operations by quickly identifying and addressing disruptions.
Cost Savings
Early detection of anomalies can lead to significant cost savings by preventing disruptions, fraud, and equipment failures. Some ways AI anomaly detection contributes to cost savings include:
- Preventing Downtime: By identifying potential equipment failures or process disruptions early, organizations can perform timely maintenance and avoid costly downtime.
- Fraud Detection: AI consulting services can detect fraudulent activities in real-time, helping to minimize financial losses and protect the organization’s assets.
- Resource Optimization: Efficient anomaly detection ensures that resources are allocated appropriately, reducing waste and improving overall operational efficiency.
Scalability
AI solutions are inherently scalable, making them suitable for organizations of all sizes and capable of handling increasing amounts of data. The scalability benefits include:
- Handling Big Data: AI models can process and analyze vast datasets efficiently, accommodating the growing data volumes generated by modern organizations. A study by IDC forecasts that AI-driven analytics and anomaly detection solutions will handle over 80% of global data traffic by 2025, showcasing their scalability.
- Flexible Deployment: AI systems can be deployed across various platforms and environments, from on-premises infrastructure to cloud-based solutions, allowing organizations to scale their anomaly detection capabilities as needed.
- Future-Proofing: The scalability of AI ensures that the anomaly detection system can evolve alongside the organization’s growth and changing data needs.
Adaptability
AI models have the ability to learn and adapt to new patterns over time, continuously improving their detection capabilities. This adaptability offers several advantages:
- Learning from Data: AI systems can be trained on new data, enabling them to recognize emerging patterns and trends that may indicate anomalies.
- Dynamic Adjustment: As AI models are exposed to more data, they can refine their algorithms and improve their accuracy in identifying anomalies. According to a study by Harvard Business Review, AI-driven anomaly detection systems improve their accuracy by 25% annually through continuous learning and adjustment.
- Resilience to Change: The adaptive nature of AI ensures that the anomaly detection system remains effective even as the underlying data and operational conditions change, providing long-term reliability.
Overview of 3 Common Anomaly Detection Methods
Anomaly detection can be broadly categorized into three methods: supervised, unsupervised, and semi-supervised. Each method has its own strengths and applications.
Supervised Anomaly Detection
Supervised anomaly detection involves training an AI model using labeled data, where anomalies are explicitly identified. The model learns to distinguish between normal and anomalous patterns based on this labeled data. This method is highly accurate but requires a substantial amount of labeled data, which can be time-consuming and costly to obtain.
Unsupervised Anomaly Detection
Unsupervised anomaly detection does not require labeled data. Instead, the AI model identifies anomalies by finding patterns that deviate from the norm within the dataset. This method is more flexible and can be applied to datasets where labeled data is unavailable. However, it may produce more false positives compared to supervised methods.
Semi-supervised Anomaly Detection
Semi-supervised anomaly detection combines elements of both supervised and unsupervised methods. It utilizes a small amount of labeled data along with a larger set of unlabeled data. This approach strikes a balance between accuracy and data requirements, making it a practical choice for many real-world applications.
Why Choose Vinova’s AI Development Services for Anomaly Detection
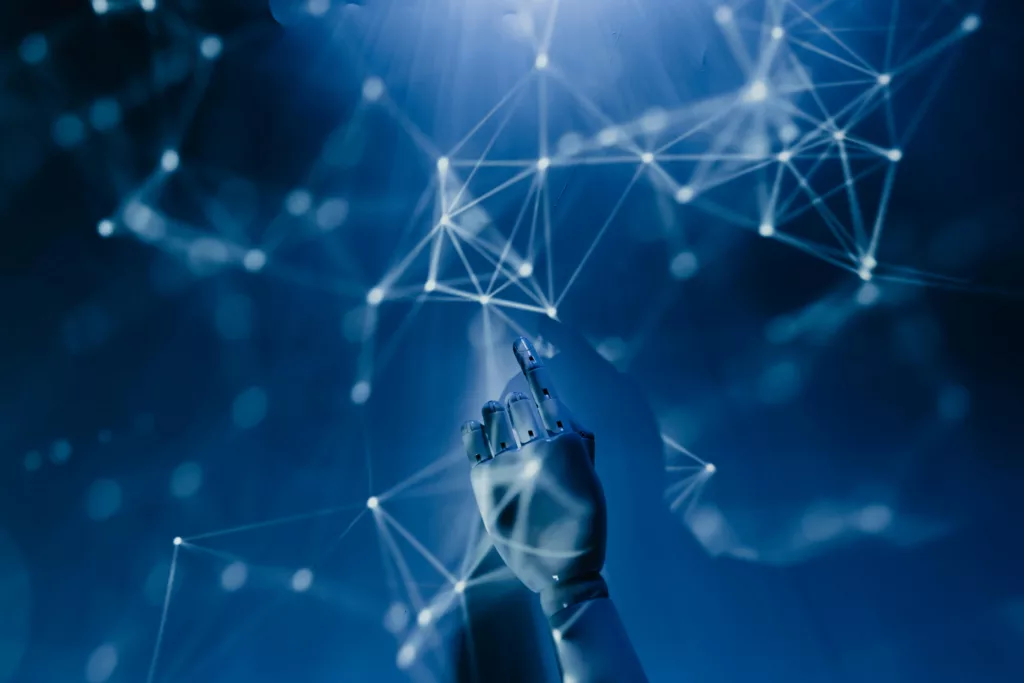
Vinova offers cutting-edge AI development services tailored to meet your anomaly detection needs. Here are some reasons why you should choose Vinova:
1. Expertise: Our team of AI experts specializes in developing and deploying cutting-edge anomaly detection solutions. With a proven track record across diverse industries, we understand the unique challenges and requirements of each sector. Our deep knowledge of AI, machine learning, and deep learning enables us to deliver accurate and reliable results.
2. Customization: Vinova provides customized AI solutions designed to address the specific challenges and requirements of your organization. Our expertise lies in developing AI algorithms that are optimized for your data patterns and anomaly types. We ensure seamless integration with your existing systems and provide scalable solutions to accommodate your growing data volumes.
3. State-of-the-art Technology: By combining machine learning and deep learning, we create intelligent systems capable of identifying complex patterns and anomalies in your data. Our solutions are designed for real-time processing, ensuring timely detection and response.
Through advanced analytics and visualization, we provide actionable insights that empower you to make informed decisions and mitigate risks effectively.
4. Comprehensive Support: From initial consultation to deployment and ongoing maintenance, Vinova offers comprehensive support to ensure the success of your AI anomaly detection project. Their end-to-end service approach guarantees that you receive the guidance and assistance needed at every stage of the project lifecycle.
5. Proven Track Record:
Vinova’s expertise in anomaly detection is backed by a strong portfolio of successful projects across diverse industries. Our commitment to client satisfaction and delivering tangible results is evident in the numerous positive testimonials and case studies.
Conclusion:
In conclusion, AI anomaly detection is a powerful tool that can enhance the efficiency and reliability of various industries by identifying and addressing irregularities in data. Whether you are in cybersecurity, finance, manufacturing, telecommunications, healthcare, or retail, AI can help you detect and respond to anomalies quickly and accurately.
By choosing Vinova’s AI development services, you can leverage the full potential of AI to protect your assets, optimize your operations, and achieve your business goals.