Artificial Intelligence (AI) has revolutionized various industries, and supply chain management is no exception. Approximately 60% of supply chain companies globally are piloting or using AI in some form
According to McKinsey, AI-driven supply chain optimization can increase warehouse productivity by 20-50%. Businesses can optimize their supply chains, enhance efficiency, and gain a competitive edge by leveraging machine learning, predictive analytics, and other AI technologies. This has propelled the usage of AI in the supply chain, as more businesses are adapting to AI.
This blog explores the transformative role of AI in supply chain management, discussing its key applications, benefits, challenges, and future trends.
Table of Contents
Understanding the Basics of AI in Supply Chain
Artificial Intelligence (AI) in supply chain management involves the use of machine learning, predictive analytics, and other AI technologies to optimize and enhance various aspects of the supply chain. Among the trends in supply chain revolutions, AI applications into supply chain is at the forefront.
By leveraging AI, companies can gain insights from large datasets, automate routine tasks, and improve decision-making processes. Understanding these basics is essential for appreciating the transformative impact of AI on supply chains.
3 key components of AI in supply chain management are:
- Machine Learning (ML): ML algorithms analyze historical data to identify patterns and demand forecasting with AI accurately. This capability allows businesses to optimize inventory levels and reduce stockouts or excess inventory.
- Predictive Analytics: Predictive analytics for the supply chain utilizes data from various sources, such as sales history, market trends, and external factors, to forecast demand. This enables companies to make informed decisions regarding production schedules and resource allocation.
- Automation: Automation in supply chain operations streamlines routine tasks within the supply chain, reducing human error and operational costs. This includes automating inventory management, order processing, and logistics operations.
Key Applications of AI in Supply Chain Management
With AI infiltrating all corners of businesses, harnessing the power of AI in supply chain management is crucial to enhance efficiency, reduce costs, and elevate service quality. Here’s how AI is transforming various aspects of the supply chain in real-world scenarios:
- Demand Forecasting: AI analyzes historical sales, market trends, and external factors to predict future demand more accurately. For instance, Amazon uses machine learning to process vast amounts of data and anticipate demand fluctuations. This helps them adjust inventory levels and minimize stockouts.
- Inventory Management: AI optimizes stock levels and reduces excess inventory. Church Brothers Farms used AI to analyze demand variables and achieved significant reductions in product wastage and improved order fulfillment rates.
- Supplier Selection and Management: AI in the supply chain helps evaluate supplier performance based on criteria like delivery times and quality. Walmart’s AI-powered processes enhance supply chain resilience by selecting suppliers that consistently meet quality standards and delivery timelines.
- Logistics and Transportation: Artificial Intelligence in logistics optimizes routing and shipping schedules to reduce delivery times and costs. Nike’s AI-driven logistics solutions analyze traffic patterns and weather conditions to adjust delivery routes, speed up deliveries and reduce fuel consumption.
- Predictive Maintenance: AI predicts equipment failures before they occur, minimizing downtime and maintenance costs. General Electric (GE) uses AI to monitor machinery health and schedule maintenance proactively, reducing unexpected downtimes.
- Quality Control: AI systems inspect products for defects and ensure high-quality standards. API Group, a printing business, employed AI for quality control and inventory optimization. This dual approach improved on-time delivery rates by 11% while reducing overstock by 8.5%.
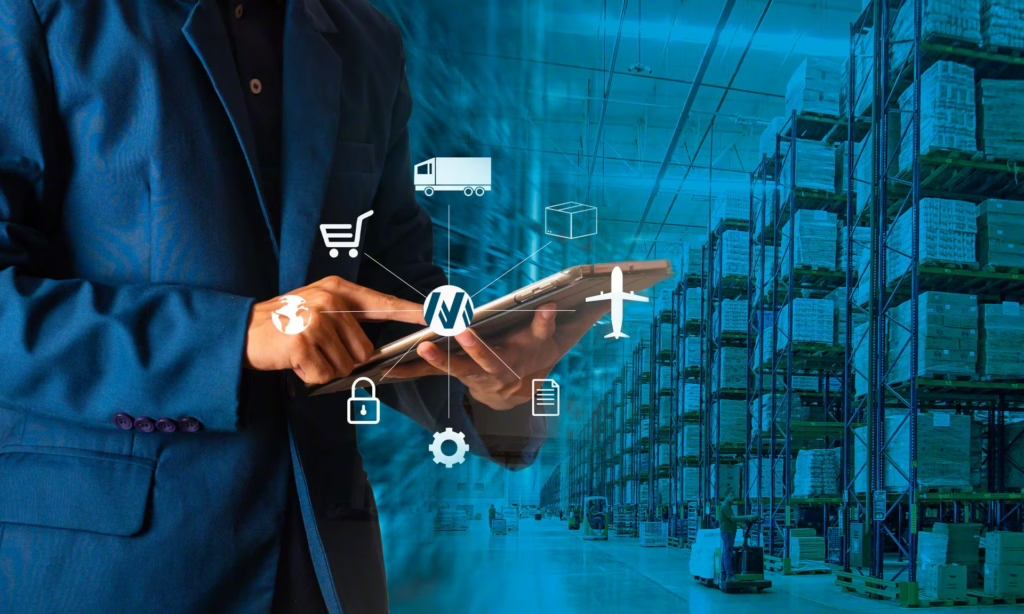
Benefits of Implementing AI in Supply Chains
The integration of AI into supply chain management is proving essential for businesses aiming to enhance operational efficiency and customer satisfaction. Here are the 5 benefits of supply chain optimization with AI:
- Increased Efficiency: Automation in supply chain operations has the potential to revolutionize the industry. By leveraging AI and robotics, routine tasks can be automated, freeing up human resources to focus on more strategic activities that require human intervention. This automation in supply chain operations can optimize routing and scheduling, resulting in significant cost reductions of up to 10-15% in transportation costs.
- Cost Reduction: Integrating Artificial Intelligence in logistics and inventory leads to significant cost savings. According to a study, AI implementations could reduce overall operational costs by 25-40%.
- Enhanced Decision-Making: 83% of supply chain executives believe that AI will enable real-time data analysis for better decision-making. This analysis provides actionable insights, improving strategic and operational decisions.
- Improved Customer Satisfaction: Faster and more accurate deliveries enhance the overall customer experience. According to a study by Boston Consulting Group, AI-driven personalization can enhance customer satisfaction by up to 30%.
- Risk Management: AI identifies potential risks and disruptions in the supply chain, enabling companies to take proactive measures. Demand forecasting with AI empowers organizations to enhance accuracy levels by 20-50%.
Challenges and Considerations in AI Adoption
The adoption of Artificial Intelligence (AI) in organizations presents several challenges that can hinder successful implementation. Understanding these challenges is crucial for businesses aiming to leverage AI effectively. Below are the primary challenges and considerations associated with AI adoption.
1. Data Quality and Availability
High-quality data is the foundation of a successful AI model. If the data is bad—inconsistent, inaccurate, or incomplete—the AI models won’t work as well as they should. Even the fanciest algorithms can’t do much with bad data, and that can negatively impact your supply chain implementation.
Getting the data ready for AI can be a lot of work, however. Sometimes, information is scattered across different systems, making it hard to put together a complete dataset to train AI models. To fix this, companies need to put money into strong data governance frameworks that make sure the data is accurate and easy to get to. These frameworks can help make the process of collecting and preparing data more efficient and less expensive.
2. Integration with Existing Systems
It can be tough to integrate AI with older systems. In our experience, many companies still use outdated tech, so old they can’t be integrated with modern AI solutions. Resolving this issue can be challenging, requiring a substantial investment of time and financial resources. You might have to redesign your whole IT system to use AI, which can slow things down and cost more.
Should you fail to successfully incorporate artificial intelligence into your supply chain infrastructure, it can lead to problems like wasted time and effort and inconsistent data. That can make it hard for AI to do what it’s supposed to do. So, it’s important to plan carefully and test everything to make sure that the AI parts work well with the systems you already have.
3. Skill Gaps
The successful implementation of AI in supply chain management requires skilled professionals conversant in AI technologies and supply chain principles. But here’s the problem: there’s a huge skills gap. A study found that 60% of companies say they can’t find people with the AI skills and training they need.
To address this challenge, organizations must prioritize upskilling their workforce through targeted training programs. That means providing targeted training programs that teach your employees about AI and supply chain management. This will help you build a team that can use AI to improve your supply chain operations and give you an edge over your competitors.
4. Cost of Implementation
When it comes to AI solutions, the upfront costs can be hefty. Organizations must balance these upfront investments against potential long-term benefits, including increased efficiency and cost savings. Many companies see the value of AI but are hesitant to commit significant resources without proof that it will pay off.
To justify the costs, companies should create detailed business cases that outline the expected benefits. Improved decision-making and operational efficiencies are major advantages of adopting AI.”
5. Ethical and Security Concerns
As organizations increasingly adopt AI technologies, addressing ethical considerations such as data privacy, algorithmic bias, and transparency becomes paramount for responsible AI use. For instance, if an AI model is trained on data that is biased, it can make decisions that are unfair or biased itself. This is because the model has learned from the biases in the data.
Also, keeping sensitive data safe is very important, especially since organizations have to deal with complicated rules like GDPR in Europe and HIPAA in healthcare. To protect user data and keep the public’s trust, there should be strong security measures and ethical rules in place.
Future Trends and Innovations in AI for Supply Chain
The integration of Artificial Intelligence (AI) in supply chain management is rapidly evolving, with several innovative trends emerging that promise to enhance efficiency, transparency, and sustainability. Below are key trends supported by real-world examples and data illustrating their impact.
1. Autonomous Vehicles and Drones
AI-powered autonomous vehicles and drones are revolutionizing transportation and delivery within supply chains. For instance, Walmart has been experimenting with autonomous delivery vehicles to streamline last-mile delivery. In a pilot program, Walmart partnered with Gatik, deploying self-driving trucks to transport goods between its dark stores and retail locations. This initiative resulted in a 30% reduction in delivery times and a significant cut in transportation costs.
Additionally, Amazon has invested heavily in drone technology for package delivery. The company aims to deliver packages weighing up to five pounds within 30 minutes using drones, which could potentially reduce delivery costs by 70% compared to traditional methods.
2. Blockchain Integration
The combination of AI with blockchain technology enhances transparency and traceability in supply chains. A notable example is the IBM Food Trust blockchain platform, which integrates AI to provide real-time tracking of food products from farm to table. By using blockchain, companies like Walmart can trace the origin of food products in seconds rather than days, significantly improving food safety and reducing waste.
In a case study involving the Thai tuna supply chain, researchers demonstrated how integrating AI with blockchain could improve compliance and traceability. The project aimed to address illegal fishing practices that cost the industry approximately $6.3 billion annually. By implementing a blockchain system alongside AI analytics, stakeholders can ensure product authenticity and enhance sustainability practices.
3. IoT and AI Collaboration
The integration of Internet of Things (IoT) devices with AI provides real-time monitoring and Predictive analytics for supply chain capabilities. For example, Siemens uses IoT sensors in its manufacturing plants to collect data on equipment performance. This data is analyzed using AI algorithms to predict maintenance needs, reducing unplanned downtime by up to 20%.
Furthermore, AI-powered inventory management systems, like the one employed by Coca-Cola, utilize IoT devices combined with AI to monitor inventory levels across vending machines. This system optimizes restocking schedules based on real-time sales data, resulting in a reported 30% reduction in operational costs.
4. AI-Powered Supply Chain Digital Twins
Creating digital twins of supply chains allows companies to simulate and optimize processes effectively. A prominent example is General Electric (GE), which uses digital twins for its manufacturing operations. By creating virtual replicas of physical assets, GE can analyze performance data and predict potential failures before they occur, thus minimizing downtime.
According to research from Deloitte, companies that implement digital twin technology can achieve up to a 25% improvement in operational efficiency by optimizing processes based on simulated scenarios.
5. Sustainability Initiatives
AI is increasingly being used to promote more sustainable supply chain practices. For instance, Unilever employs AI-driven analytics to optimize its logistics network, resulting in a 10% reduction in carbon emissions over three years. The company utilizes machine learning algorithms to analyze transportation routes and minimize fuel consumption effectively.
Additionally, the integration of AI with renewable energy sources is gaining traction; companies like Tesla use AI algorithms to manage energy consumption across their supply chains, aiming for carbon neutrality by 2030.
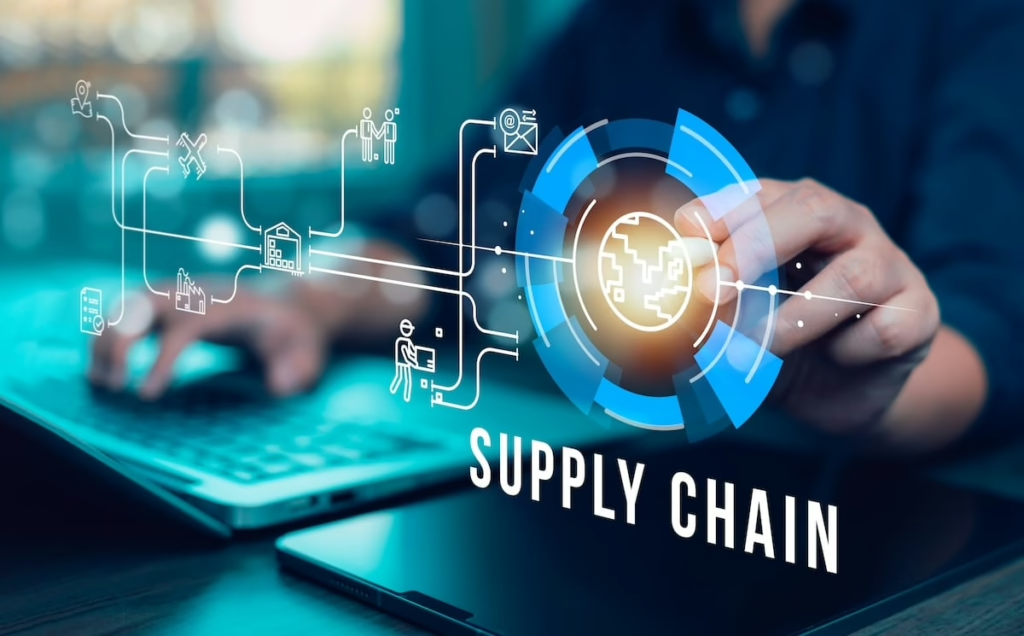
Conclusion
The integration of artificial intelligence (AI) in supply chain management can lead to increased efficiency, transparency, and sustainability. AI-powered systems can analyze vast amounts of data, identify patterns, and make predictions, enabling businesses to make data-driven decisions, streamline operations, and gain a competitive edge.
Don’t let outdated processes and inefficiencies hold your supply chain back.
At Vinova, we believe that AI has the power to transform supply chains, making them more efficient, transparent, and sustainable. From demand forecasting and inventory management to logistics and sustainability, we have the experience and knowledge to help you succeed, our team of experts will work with you to identify areas for improvement and develop a customized AI solution that meets your unique needs.
Join Vinova on the journey to supply chain excellence with AI. Contact us today to schedule a consultation and learn how we can help you unlock the full potential of your supply chain.
Frequently Asked Questions
What is AI in supply chain management?
AI in supply chain management refers to the utilization of artificial intelligence technologies to enhance and optimize various aspects of the supply chain, including demand forecasting, inventory management, and logistics. AI-powered systems can analyze vast amounts of data, identify patterns, and make predictions, enabling businesses to make data-driven decisions, streamline operations, and gain a competitive edge.
How does AI improve demand forecasting?
AI plays a crucial role in enhancing demand forecasting accuracy. Traditional methods often rely on historical data and simple statistical models, which may not capture the complexity and dynamism of the market. AI, on the other hand, can analyze vast amounts of structured and unstructured data, including social media sentiment, weather patterns, economic indicators, and competitor activities. By leveraging machine learning algorithms, AI systems can identify hidden patterns and relationships, allowing businesses to make more accurate predictions about future demand.
What are the benefits of AI in logistics?
AI-powered inventory management transforms the logistics sector, enabling efficient and accurate stock control. Through real-time data analysis from sensors, GPS, and historical records, AI optimizes inventory levels, forecasts demand patterns, and streamlines order fulfillment processes. This advanced system minimizes stockouts, reduces overstocking costs, and ensures optimal inventory allocation across warehouses and distribution centers. AI-powered inventory management empowers logistics providers to make proactive decisions, adapt to market fluctuations, and deliver exceptional customer service by providing real-time inventory visibility and predictive insights.
What are the challenges of implementing AI in supply chain management?
While AI holds great promise for supply chain management, certain challenges need to be addressed for successful implementation. These include:
- Data quality: AI algorithms rely on high-quality data for accurate predictions. However, supply chain data can often be fragmented, inconsistent, and incomplete.
- Integration with existing systems: Implementing AI solutions may require integration with existing supply chain systems, which can be complex and time-consuming.
- Skill gaps: The adoption of AI requires a workforce with specialized skills in data science, machine learning, and supply chain management.
- Cost of implementation: Investing in AI technology and infrastructure can be expensive, particularly for small and medium-sized businesses.
- Ethical concerns: The use of AI raises ethical questions related to data privacy, algorithmic bias, and the impact on employment.
What is the future of AI in supply chain management?
The future of AI in supply chain management is promising and characterized by several emerging trends:
- Autonomous vehicles: AI-powered autonomous vehicles will revolutionize transportation and logistics, enabling faster, more efficient, and safer deliveries.
- Blockchain integration: Blockchain technology can be integrated with AI to enhance traceability, transparency, and security in the supply chain.
- IoT collaboration: AI will work in conjunction with IoT devices to monitor inventory levels, track asset locations, and optimize warehouse operations.
- AI-powered digital twins: Digital twins, virtual replicas of physical supply chain systems, will be enhanced with AI to simulate scenarios, test strategies, and make real-time decisions.
- Sustainability initiatives: AI will play a vital role in promoting sustainability in the supply chain, optimizing energy consumption, reducing waste, and supporting circular economy models.