Table of Contents
A reflection on my own data science self-study journey
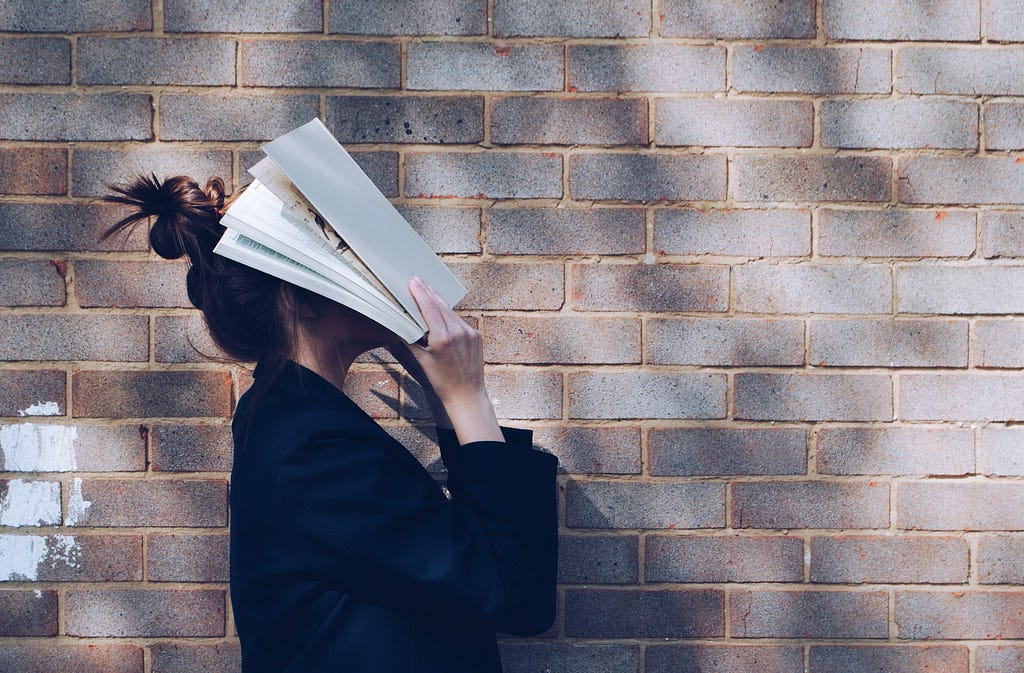
Self-study has become an increasingly popular and viable route to take to enter the field of data science. However, like in any field, self-study comes with a lot of challenges — you have to build your own curriculum, keep yourself motivated and hold yourself accountable for your learning. I was no exception to these challenges and I made a lot of mistakes on my journey.
I created a 6-month data science self-study curriculum that started in November 2021. Even though I had already obtained a Master’s degree in Statistics and worked in data science for a few years, the truth is that data science is advancing rapidly and there was still so much I didn’t know.
After reading about Daniel Bourke’s AI masters curriculum, I thought it would be a great idea to create a curriculum of my own to build and improve my data science skills.
However, my 6-month self-study curriculum did not go as planned. If you’re thinking of self-studying data science then I encourage you not to make the same mistakes I did.
My data science curriculum
The curriculum I created had a mix of courses and books. I tried to incorporate resources from different sources so that I was not only relying on a single person/company/platform. If one resource did not explain things in a way that helped me then I could easily switch to another than taught the material in a way that I understood.
Foundations & Machine Learning:
Deep Learning:
Additional Resources:
I completed around half of the material I intended to cover in my curriculum. I made a lot of mistakes in my self-study journey, and I’d like to share them in this article.
Mistake #1: Worrying too much about the best course or the best book to learn data science
We are really spoiled for choice with the number of books, courses, YouTube videos, blog posts, and so on that are out there to learn data science. It can be difficult to choose the ones you will use in your own learning and so naturally, you’d want to only use the best or most recommended resources.
Preparation is very important for self-study. Scott Young states in his book Ultralearning that around 10% of your total investment in the project should be spent on ‘meta learning’ or preparation for what you will learn and how.
I overlooked my own learning style when doing this preparation and put too much emphasis on a resource that was highly recommended when it actually was not right for me. The truth is that there is just no such thing as a perfect course or a perfect book and I learnt this the hard way.
Mistake #2: Using resources that did not match my learning style
I do not learn well from video courses or lectures. You would think that I’d have learnt my lesson from the many years I spent in university, but no, I did not. I learn best with highly active methods. I cannot learn passively, it just does not work for me.
In university, the number 1 thing that got me to graduate summa cum laude was the copious amounts of practice questions, past exam papers, exercises, and project assignments I did. I retained very little information from sitting in a lecture, whether I took notes or not. I needed to actually engage with the material in an active way to be able to remember or learn anything.
This was no different for my self-study journey. I realised that all the video courses I had planned in my curriculum were not helping me to learn — they were too passive. Because of this, I would go down many rabbit holes trying to understand concepts that were discussed in the video course and would be left feeling very frustrated.
Mistake #3: Not doing enough projects
My intention with my curriculum was to do as many projects as possible as I progressed through the various concepts and methods I was learning. This started out very well with the Hands on Machine Learning book and I did a project for each chapter but this changed when I started doing the video-based courses — I entered passive mode.
Tina Huang mentions in one of her YouTube videos that you should only learn as much as you need so that you can start applying your knowledge in a project. This is actually the most important part of studying data science — projects, projects, projects!
Mistake #4: Fixating on learning ALL the math
Machine learning is built on a foundation of mathematics. However, you do not need to know or understand ALL the math in order to learn, apply or do data science. During my self-study journey, I fixated too much on understanding each mathematical concept before progressing to the next topic.
This is something that was ingrained into me from my university days. Proving theorems and working out algorithms by hand were standard in the learning process. While I do believe that having some mathematical foundation is important to get a deep understanding of a concept, it definitely is not necessary to know or understand every mathematical concept used in a method. It simply does not inherently make you a better data scientist.
The most important factor in doing a good job as a data scientist (in my opinion) is problem-solving. Can you take a problem that is presented to you and formulate a solution that will be valuable to the business?
Mistake #5: Reading research papers too soon in my journey
Whether or not to read research papers is a tricky topic in data science and it can be very overwhelming given how much new research is published on a daily basis.
Before starting this self-study journey, I did not know much at all about deep learning. I was a complete beginner. I made the mistake of thinking that I needed to read (and understand) the research papers that were associated with some of the topics I was learning.
Reading research papers before I had a solid understanding of the surrounding concepts and methods only made me feel frustrated and inadequate like I was somehow a ‘bad’ data scientist because these research papers made very little sense to me.
It is not necessary to read research papers in your learning journey, especially if you are still new to the material. If you are interested in research papers, I do recommend leaving them for much later on when your foundations are solid.
What’s next?
My self-study journey is not over simply because the 6-month timeframe is up. Learning is a constant part of being a data scientist and I thoroughly enjoy it.
If anything, my mistakes and failures have taught me what not to do, and serve as a guide for my future learning goals. Going forward, I will be focussing on 2 main aspects of my learning:
- Using resources that involve more active forms of learning
- Doing more projects
For the first point, I have found an excellent online book (that’s free) called Dive Into Deep Learning that I have already been using heavily to study deep learning. It covers each topic with a mix of math, code, and explanations and encourages project-based learning with real datasets.
I am using DataCamp Workspaces to find data and complete projects for the second point. The reason I am using DataCamp Workspaces is that every dataset they offer has a challenges and scenarios section. These are very helpful when you don’t know where to start with a new dataset.
I find the scenarios to be particularly helpful since they place you in a problem-solving mode right away. Using this you can build an end-to-end project for your portfolio that showcases more than just your knowledge of machine learning models but also the business and problem-solving aspects too.
Conclusion
I hope that sharing the mistakes I made in this article can help you in your self-study journey.
At the end of the day, self-study is as much about exploring yourself as it is about learning the material. You learn what works for you and what doesn’t and sometimes that’s not going to match up with everybody else’s processes or recommendations and that’s ok.
Above all, data science is not a spectator sport — eventually you will need to get your hands dirty doing projects and implementing the things you learn even if you don’t feel ‘ready’ yet.
Do You Want to Self-Study Data Science? Learn From My Mistakes was originally published in Towards Data Science on Medium, where people are continuing the conversation by highlighting and responding to this story.
This content was originally published here.