Multimodal AI is a groundbreaking technology that combines multiple modalities, such as text, images, and audio, to create more sophisticated AI applications. With over 1.5 million users, DALL-E, a multimodal generative AI from OpenAI, demonstrates the power of multimodal AI in generating creative content.
In this blog post, we’ll explore 10 creative applications of multimodal AI and how it’s transforming industries. From personalized healthcare to empathetic retail assistants, multimodal AI is revolutionizing the way we interact with technology.
Table of Contents
What is Multimodal AI?
Multimodal AI is a powerful technology that combines multiple types of data, such as text, images, speech, and video, into a single AI system. This fusion allows for a deeper understanding of context and enables AI to perform tasks that were previously thought to be beyond its capabilities.
Key points about multimodal AI:
- Combines Modalities: Multimodal AI algorithms can process and interpret information from various sources simultaneously, providing a richer understanding of context.
- Contextual Understanding: By considering multiple modalities, multimodal AI can achieve a deeper level of contextual understanding, which is crucial in tasks like natural language understanding and human-computer interaction.
Example:
Consider a social media platform where users upload photos with captions. A multimodal AI system can analyze both the image and the text to generate more accurate and contextually relevant descriptions. This is known as “multimodal machine learning”. For instance, if a user posts a picture of a serene beach at sunset with the caption “Relaxing day at the beach,” the AI can combine the visual and textual information to generate a more descriptive caption like “Golden hues paint the tranquil beach as the sun bids farewell.”
By leveraging the power of multiple modalities, multimodal AI opens up new possibilities for AI applications across various industries.
Top Benefits of Multimodal AI for Businesses
A multimodal AI application offers businesses a range of advantages by combining multimodal models to gain deeper insights and improve decision-making.
Enhanced Decision Making
- Informed Decisions: By integrating diverse data sources, multimodal AI enables businesses to make more informed and accurate decisions.
- Example: Northumbria Healthcare NHS Foundation Trust used multimodal AI to improve diagnostic accuracy by combining medical images with patient records.
- Impact: Radiologists could analyze both visual evidence and relevant patient history, leading to better diagnoses and improved patient outcomes.
Improved User Experience
- Personalized and Intuitive Interactions: Applications that understand both text and visual cues can provide a more tailored and engaging user experience.
- Example: Corpora Studios implemented multimodal AI in their virtual assistant to understand both voice commands and textual queries, providing personalized responses and visual information.
- Impact: Users benefit from a more intuitive and satisfying experience, as they can interact with the assistant using their preferred method.
Robustness and Adaptability
- Resilience to Missing or Noisy Data: Multimodal models can handle incomplete or inaccurate data from one modality by relying on information from others.
- Example: CarMax uses AI consulting services for inventory management, combining textual descriptions with vehicle images. Even with incomplete or noisy descriptions, the system can rely on visual data to ensure accurate listings.
- Impact: Businesses can maintain the reliability and accuracy of their applications, even in the face of imperfect or incomplete data.
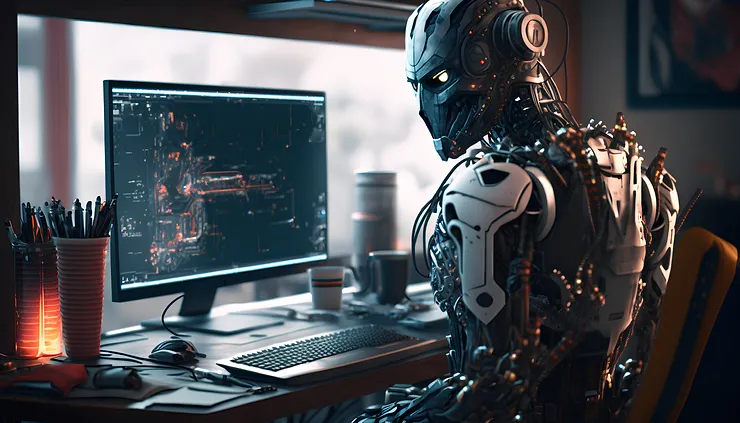
How Does Multimodal AI Work?
Multimodal AI systems utilize deep learning architectures to process and integrate information from multiple modalities. Here’s a simplified breakdown of the key steps involving multimodal models:
Feature Extraction
- Breaking Down Modalities: Each modality (text, image, audio) is processed using specialized techniques to extract relevant features.
- Text: Word embeddings are used to convert words into numerical representations that capture their meaning.
- Images: Convolutional neural networks (CNNs) are employed to extract visual features such as edges, textures, and shapes.
Fusion Techniques
- Combining Modalities: The extracted features from different modalities are combined to create a unified representation.
- Concatenation: A simple approach is to concatenate the feature vectors from each modality.
- Attention Mechanisms: These mechanisms allow the model to focus on the most relevant parts of each modality, ensuring that the fusion process is effective.
- Joint Embeddings: This technique translates different modalities into a shared space, enabling the model to process and understand information from various sources.
Training the Multimodal Model
- Data Preparation: A large dataset of paired examples (e.g., text and images) is required to train the multimodal model.
- Loss Function: The model’s parameters are optimized by minimizing a loss function, which measures the difference between the model’s predictions and the ground truth.
- Backpropagation: This technique is used to adjust the model’s weights and biases to improve its performance, aka improving an AI’s multimodal machine learning.
Predictions and Adaptability
- Inference: Once trained, the multimodal model can be used to make predictions based on new input data.
- Robustness: Multimodal models are often more robust than unimodal models because they can rely on other modalities if data from one source is missing or noisy.
By following these steps, multimodal generative AI systems can effectively process and integrate information from various sources, enabling them to perform complex tasks and make informed decisions.
Real-World Applications of Multimodal AI
Let’s explore how multimodal AI is making an impact across various domains, complete with multimodal AI examples for better demonstrations:
Healthcare
Medical Imaging
- Enhanced Disease Detection: By integrating radiology images with patient history, multimodal AI can improve the accuracy of disease detection.
- Example: AI-enabled camera technology in CT scans can automatically detect anatomical landmarks, ensuring accurate patient positioning and reducing radiation dose.
- Benefit: Improved image quality, streamlined workflows, and enhanced diagnostic confidence.
Health Monitoring
- Personalized Health Insights: Wearable devices that combine sensor data with contextual information can provide personalized health insights.
- Example: Apple Watch and Fitbit use multimodal AI to analyze heart rate, activity levels, and conduct ECG readings.
- Benefit: Real-time health monitoring, detection of irregular heart rhythms, and early identification of potential health issues.
By leveraging multimodal AI, healthcare providers can:
- Improve diagnostic accuracy
- Enhance patient care
- Enable personalized health monitoring
- Reduce costs
- Streamline workflows
Finance
Fraud Detection
- Identifying Unusual Activities: By combining transaction data, user behavior, and text-based communication, multimodal AI can effectively identify fraudulent activities.
- Example: JPMorgan Chase uses anomaly detection algorithms to flag transactions that deviate from a customer’s typical behavior, improving fraud detection rates.
- Benefit: A more secure banking experience and reduced financial losses due to fraudulent activities.
Sentiment Analysis
- Informed Investment Decisions: Analyzing financial news articles alongside market data can help investors make more informed decisions.
- Example: Financial institutions use multimodal AI to understand market sentiment by analyzing news articles and market data.
- Benefit: By identifying trends and potential risks, investors can adjust their investment strategies accordingly, potentially leading to higher returns.
In summary, multimodal AI is revolutionizing the financial industry by:
- Enhancing fraud detection
- Improving investment decision-making
- Providing valuable insights for financial institutions
E-commerce
Visual Search
- Enhanced Product Discovery: Visual search allows users to find products by uploading images instead of relying solely on keywords.
- Example: Pinterest Lens lets users photograph an item and find similar products on the platform.
- Benefit: Simplifies the shopping process and helps users discover products they might not have found otherwise.
Product Recommendations
- Personalized Recommendations: By combining browsing behavior, purchase history, and product images, multimodal AI can provide highly personalized product recommendations.
- Example: Amazon’s recommendation engine suggests related or complementary products based on user preferences and purchase history.
- Benefit: Increased sales, improved customer satisfaction, and enhanced customer engagement.
In summary, multimodal AI is revolutionizing e-commerce by:
- Enhancing product discovery
- Providing personalized recommendations
- Improving the overall shopping experience
- Driving sales and customer loyalty
Education
Automated Grading
- Efficient and Consistent Grading: Automated grading systems can evaluate student assignments using both text and visual cues, saving educators time and ensuring consistent assessment.
- Example: Gradescope has reduced grading time by 70% by using AI to assess assignments.
- Benefit: Allows educators to focus more on teaching and providing personalized support to students.
Personalized Learning
- Tailored Content: Multimodal AI can adapt educational content to individual student preferences and learning styles.
- Example: Duolingo uses multimodal AI to personalize language lessons based on user progress, preferences, and learning styles.
- Benefit: Enhances student engagement, retention, and overall learning outcomes.
In summary, multimodal AI is revolutionizing education by:
- Automating grading tasks
- Providing personalized learning experiences
- Improving efficiency and effectiveness
Manufacturing
Quality Control
- Enhanced Product Quality: By combining visual data with sensor readings, multimodal AI can detect even the smallest defects and anomalies in products.
- Example: Automated visual inspection systems can analyze images and sensor data to identify surface imperfections, misalignments, and faulty components.
- Benefit: A significant reduction in defects, improved product consistency, and increased production efficiency.
Predictive Maintenance
- Reduced Downtime: By combining sensor data, maintenance logs, and images, multimodal AI can predict equipment failures before they occur.
- Example: Semiconductor manufacturers use multimodal AI to analyze sensor data, maintenance logs, and microscopic images to predict equipment breakdowns.
- Benefit: Early warnings allow maintenance teams to perform targeted maintenance, preventing costly downtime and reducing maintenance costs.
In summary, multimodal AI is revolutionizing manufacturing by:
- Improving product quality
- Enhancing efficiency
- Reducing costs
- Increasing competitiveness
Agriculture
Crop Monitoring
- Precision Agriculture: By combining satellite imagery, weather data, and soil information, multimodal AI enables farmers to optimize crop management practices.
- Example: Analyzing satellite imagery and weather data can help detect crop stress, optimize irrigation schedules, and predict yield variations.
- Benefit: Improved crop yields, reduced resource consumption, and increased sustainability.
Livestock Health
- Early Disease Detection: Integrating visual cues with health records allows for early detection of diseases and improved animal welfare.
- Example: AI-based sensors can monitor individual animals in real-time, capturing visual cues and health data.
- Benefit: Reduced mortality rates, optimized feeding schedules, and overall improved herd health.
In summary, multimodal AI is revolutionizing agriculture by:
- Enhancing crop management
- Improving livestock health
- Increasing sustainability
- Improving efficiency and productivity
Retail
Visual Merchandising
- Optimized Store Design: By analyzing customer movement patterns captured by cameras, retailers can optimize store layouts to improve customer experience and increase sales.
- Example: Heat maps generated from computer vision data can reveal which store areas receive the most foot traffic, allowing retailers to strategically place high-demand items.
- Benefit: Improved sales conversion rates, better utilization of store space, and enhanced customer satisfaction.
Checkout-Free Stores
- Seamless Shopping Experience: Combining computer vision with transaction data enables retailers to create checkout-free stores, eliminating the need for traditional checkout lines.
- Example: Amazon Go stores use a combination of technologies to track customer purchases and automatically charge their accounts.
- Benefit: Reduced waiting times, improved customer satisfaction, and increased operational efficiency.
In summary, multimodal AI is revolutionizing retail by:
- Enhancing visual merchandising
- Enabling checkout-free shopping
- Improving customer experience
- Increasing operational efficiency
Consumer Technology
Smart Assistants
- Enhanced User Interactions: By combining voice commands with visual context, multimodal AI enables smart assistants to provide more relevant and informative responses.
- Example: Google Assistant can understand both spoken queries and visual information, providing a more natural and seamless user experience.
- Benefit: Improved user satisfaction, engagement, and overall experience.
AR/VR
- Immersive Experiences: Multimodal AI is essential for creating immersive AR/VR experiences by combining real-world visuals with virtual overlays.
- Example: Microsoft HoloLens uses multimodal AI to overlay virtual objects onto the real world, creating a mixed-reality environment.
- Benefit: Increased productivity in various fields, including architecture, manufacturing, and education.
In summary, multimodal AI is revolutionizing consumer technology by:
- Enhancing smart assistant interactions
- Creating immersive AR/VR experiences
- Improving user satisfaction and engagement
Energy
Predicting Energy Demand
- Enhanced Grid Management: By analyzing historical usage patterns and weather data, AI models can accurately predict future energy demand.
- Example: Utility companies can use multimodal AI to optimize energy supply and reduce waste.
- Benefit: Improved grid stability, cost savings, and enhanced energy management.
Infrastructure Monitoring
- Early Detection of Defects: Combining sensor data and visual inspections allows for early detection of potential failures in energy infrastructure.
- Example: Drones can be used to inspect transmission lines for wear and corrosion, while AI algorithms analyze sensor data to identify anomalies.
- Benefit: Reduced downtime, improved safety, and more cost-effective maintenance.
In summary, multimodal AI is revolutionizing the energy sector by:
- Improving energy demand forecasting
- Enhancing infrastructure monitoring
- Increasing efficiency and sustainability
Social Media
Content Moderation
- Detecting Harmful Content: By analyzing both text and images, multimodal AI can effectively detect harmful content such as hate speech, harassment, and inappropriate material.
- Example: Social media platforms use AI-powered systems to identify and remove harmful content, improving user safety and reducing the workload for human moderators.
- Benefit: Faster response times, improved user safety, and more effective enforcement of community guidelines.
Personalized Feeds
- Tailored Content Discovery: Multimodal AI can tailor content feeds to individual user preferences and interests.
- Example: TikTok’s For You Page (FYP) uses advanced algorithms to curate personalized video recommendations based on user behavior and preferences.
- Benefit: Increased user engagement, longer time spent on the platform, and a more enjoyable user experience.
In summary, multimodal AI is revolutionizing social media by:
- Improving content moderation
- Enhancing user experience
- Creating safer and more personalized online environments
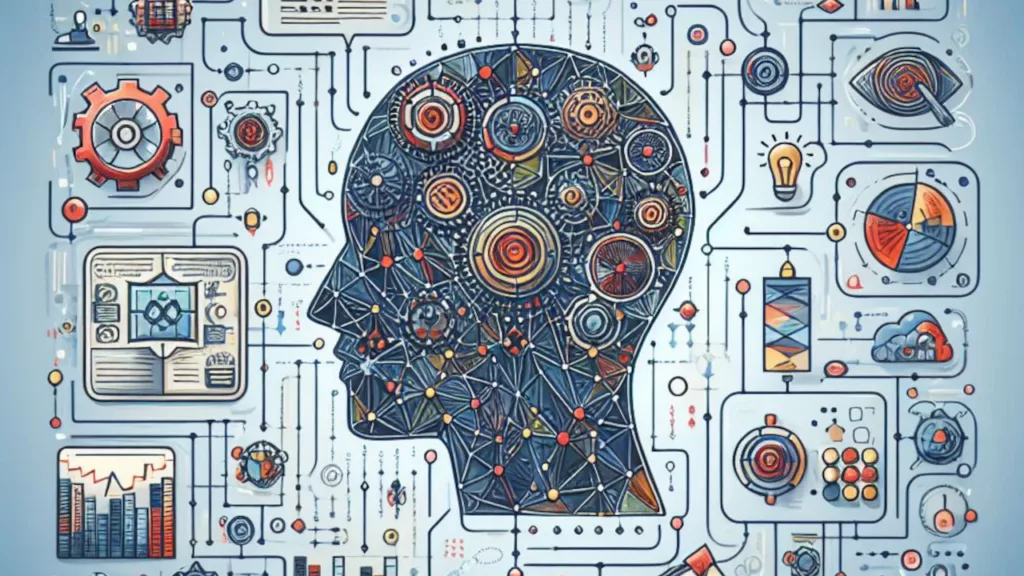
Potential Risks of Implementing Multimodal AI
While multimodal AI offers numerous benefits, it’s essential to address the potential risks and challenges associated with its implementation:
Data Privacy
- Robust Measures Required: Handling diverse data modalities, including text, images, and other sensitive information, requires stringent privacy measures.
- Compliance: Businesses must ensure compliance with relevant data privacy regulations, such as GDPR and CCPA. This involves anonymizing data, securing storage and transmission, and obtaining informed consent from users.
Complexity
- Intricate Development: Developing and maintaining multimodal models can be complex, as they often involve multiple neural networks specialized in processing different modalities.
- Resource-Intensive: Ensuring seamless fusion of these modalities during training and inference can be computationally demanding.
Scalability
- Computational Resources: Training large-scale multimodal models requires significant computational power.
- Deployment Challenges: Deploying such models across distributed environments can be complex and requires careful orchestration.
By addressing these potential risks and challenges, businesses can effectively harness the power of multimodal AI while minimizing its drawbacks.
Key Differences Between Generative AI, Unimodal AI, and Multimodal AI
Generative AI, unimodal AI, and multimodal AI each have their unique characteristics and applications.
- Generative AI focuses on creating new content, such as images, text, or music, using models like GANs.
- Unimodal AI deals with a single modality of data, like text or images, and is often used for tasks like sentiment analysis or image classification.
- Multimodal AI integrates multiple modalities, allowing for a richer understanding of context and enabling more complex applications.
In summary:
- Generative AI: Creates new content.
- Unimodal AI: Deals with a single modality.
- Multimodal AI: Combines multiple modalities for a richer understanding.
By understanding these distinctions, businesses can choose the most appropriate type of AI to meet their specific needs and goals.
Conclusion
Staying informed about the latest advancements is crucial. Explore our blog for in-depth articles, tutorials, and thought-provoking discussions on various AI topics, including machine learning, natural language processing, and multimodal AI. Discover real-world applications and learn how to harness the power of AI for your own projects.
Need expert advice? Our team of AI experts is here to help. Whether you have a specific question or need personalized guidance, we’re ready to assist you. Contact us today and let’s embark on your AI journey together.